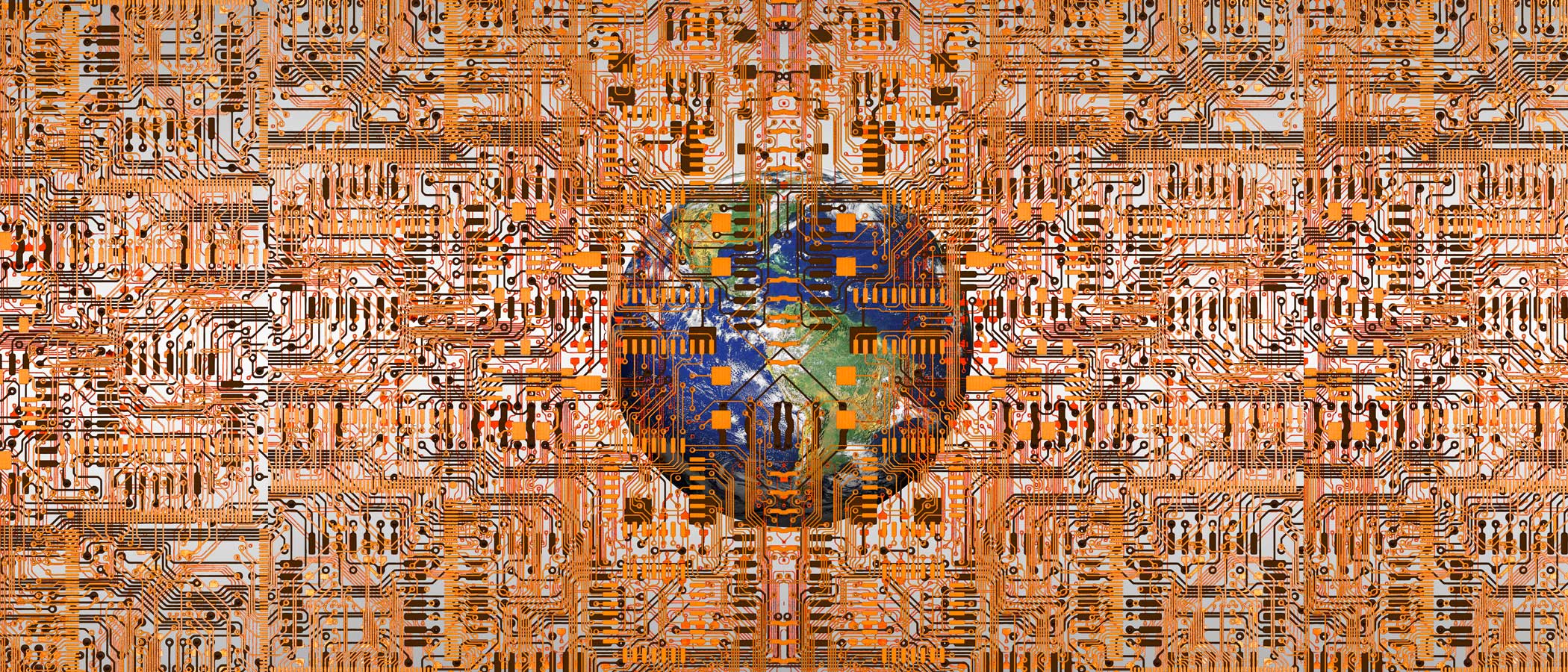
The generative world order: AI, geopolitics, and power
Jared Cohen is president of Global Affairs and co-head of the Office of Applied Innovation.
George Lee is co-head of the Office of Applied Innovation.
Lucas Greenbaum, Frank Long, and Wilson Shirley are contributors.
Executive summary
- The emergence of generative AI marks a transformational moment that will influence the course of markets and alter the balance of power among nations. Increasingly capable machine intelligence will profoundly impact matters of growth, productivity, competition, national defense and human culture. In this swiftly evolving arena, corporate and political leaders alike are seeking to decipher the implications of this abrupt and powerful wave of innovation, exploring new opportunities and navigating new risks.
- The world is facing a narrow window of opportunity – what we call the inter-AI years – to shape the AI-enabled future. This window will be brief – a few years at most – then views and strategies will harden; norms, values, standards will be embedded within the technology; and the costs of changing course will rise. While AI-enabled technology will continue to progress, decisions made today will determine what is possible in the future. A generative world order will emerge.
- The US and China are the world’s top AI competitors, but they are also the top AI collaborators. However, in generative AI, most of the cutting-edge innovations today are coming from the US, and China faces an uphill in training LLMs, for now. This technology competition will see geopolitical priorities drive economic decision-making, including through export controls, sanctions, tariffs, industrial policy, investment screening, and other measures deployed to increase absolute and relative advantages.
- Geopolitical swing states will have a meaningful role in shaping the AI-enabled future. In particular, UK, the UAE, Israel, Japan, the Netherlands, South Korea, Taiwan, and India are the key players in the category. Moreover, these players may form innovation blocs, creating alliances and partnerships with more dominant states or cooperating with each other to pursue common goals.
- The technology’s development will shape its geopolitical effects. New capabilities will lead to novel use cases and broader impact. We expect generative AI models to become increasingly multimodal, seamlessly reckoning with text, images, audio and video. Focus will also shift toward performance and value engineering, making this technology less costly and more sustainable. The incorporation of persistent memory will enable better long-range planning and true agentic scenarios. Perhaps the timeliest question is whether generative AI will follow a scale up trajectory, with models increasing in size and likely dominated by large commercial enterprises, or whether they will scale down, favoring a broader set of providers and open source approaches.
- The most profound impact of generative AI may be on economic growth. Goldman Sachs Research estimates a baseline case in which the widespread adoption of AI could contribute 1.5% to annual productivity growth over a ten-year period, lifting global GDP by nearly $7 trillion. The upside case carries a remarkable 2.9% total uplift. But these outcomes are not guaranteed and will be determined by four key components: energy, compute, data, and models.
- Next year will witness several key milestones for the future of generative AI. The world’s three largest democracies, and approximately 41% of the global population, will participate in national elections. AI will continue to accelerate and be adopted by state and commercial actors for everything from defense, to health care, to education, and more. At the end of 2024, we will have a better idea how AI will transform scientific discovery, labor, and the balance of power.
Introduction
Escalating competition between the US and China, wars in Europe and the Middle East, and shifting global alliances have ushered in the most unstable geopolitical period since the Cold War. At the same time, we are experiencing what may be the most significant innovation since the internet: the rise of generative artificial intelligence. With the public release of ChatGPT on November 30, 2022, the defining geopolitical and technological revolutions of our time collided.
Over the last 20 years, “traditional AI” has become pervasive, powering advertising algorithms, content recommendations, and social-media targeting. But its power and influence were largely shrouded and embedded within applications. Now, generative AI is front-and-center, with user interfaces that are commonplace, proficient, and clearly identifiable as machine-driven intelligence. Unlike previous technological revolutions, from the printing press to the internet, leaders in every capital and commercial center gained access to this tool at the same time, as ChatGPT became the fastest-adopted technology in history. While the technology’s future is uncertain, generative AI was nearly universally acknowledged as a paradigm-shifting innovation, not a fleeting trend or hype cycle. With widespread adoption and accelerating innovation, we have now entered a period we call the inter-AI years, when leaders in every sector are working to understand what generative AI will mean for them, and how they can take advantage of opportunities while mitigating risks.
This simultaneity does not imply parity. Different countries and companies have diverse histories, contexts, capabilities, and risk tolerance when it comes to AI. Notwithstanding these differences, leaders have the same window of maximum flexibility to shape an AI-enabled future. This window will be brief – a few years at most – then views and strategies will harden, and norms, values, and standards will be embedded within the technology. While the technology will continue to progress, decisions made today will determine what is possible in the future. Countries will have devised their approaches and formed likeminded blocs, competition will increase, the costs of changing course will rise, and the available paths for companies and countries will be more determined. What will emerge is a generative world order.
We are at the beginning of that order, and its future is uncertain. The long-term effects of technological revolutions are not made clear overnight – the Reformation did not immediately follow the invention of the printing press, and few could have predicted the positive and negative effects of social media in the early 2000s. But before long, the way we live and the nature of global politics will be shaped by generative AI. Which winners and losers will emerge? Will generative AI prove to be a zero-sum game, or create win-win scenarios? Will the problems that generative AI creates be solved by the very same technology, or will they prove to be intractable? Will companies or governments drive the future of AI? The relative nascency of this new node of AI innovation and the steep trajectory of improvement in generative AI models make these questions more momentous, and difficult to answer.
We believe that this is the time to take stock of where we are, and to identify some of the inflection points that will shape our AI future. This paper takes up the key changes in the generative AI field over the last year in the domains of geopolitics, technology, and markets. We discuss what trends are emerging, what debates remain unsettled, and how the generative world order is being defined.
AI and geopolitics
Geopolitical competition is a constant. But the technologies that animate that competition are not. And while the United States, China, and Russia do not agree on many things, they all acknowledge that AI could reshape the balance of power.
Today’s geopolitical rivals are putting AI at the center of their national strategies. In 2017, Russian President Vladimir Putin said, “Artificial intelligence is the future not only of Russia but of all of mankind.” Five years later, Chinese Communist Party General Secretary Xi Jinping declared, “We will focus on national strategic needs, gather strength to carry out indigenous and leading scientific and technological research, and resolutely win the battle in key core technologies.” And, in 2023, US President Joe Biden summarized what AI will mean for humanity: “We’re going to see more technological change in the next 10 – maybe the next 5 years – than we’ve seen in the last 50 years…Artificial intelligence is accelerating that change.”
Today, there are two AI superpowers, the United States and China. But they are not the only countries that will define the technology’s future. Earlier this year, we identified a category of geopolitical swing states – non-great powers with the capacity, agency, and increasingly the will to assert themselves on the global stage. Many of these states have the power to meaningfully shape the future of AI. There are also emerging economies that have the potential to reap the rewards of AI – if the right policies and institutions are established – and whose talent, resources, and voices are essential to ensure that the creation of a human-like intelligence benefits all of humanity.
The AI incumbents: The US & China
The US and China are the world’s leading AI competitors, but they are also the most important AI collaborators. According to Stanford University’s 2023 Artificial Intelligence Index Report, despite increased geopolitical competition, the number of AI research collaborations between the two countries quadrupled between 2010 and 2021, though the rate of collaboration has slowed significantly since then, and will likely continue to do so. More so every day, AI is a critical domain in which the US and China cooperate, compete, and confront one another economically, technologically, politically, and militarily.
The great power AI competition focuses on hardware, data, software, and talent. Each country is pushing the technology further, developing AI champions, and finding the most relevant use cases and advantageous areas for AI adoption. The US and China are, in different ways, seeking to advance their absolute and relative positions, protect their interests, and secure leverage as they each follow distinct strategies.
The US is the world’s preeminent AI power, thanks to its world-leading universities and companies. Working alongside a global network of allies and partners on everything from research to export controls, Washington is concerned with keeping its advantages and accelerating the pace of domestic AI innovation. American industry leaders warn of the potential perils if the US were to “slow down American industry in such a way that China or somebody else makes faster progress.” Meanwhile, China will continue executing its long-term, state-led policies, including initiatives like “Made in China 2025” to increase self-reliance, bolster its domestic AI industry, and increase its leverage over competitors.
Today, large language models (LLMs) are the primary arena of AI innovation and competition, and countries are racing to develop more advanced and capable LLMs. In general, LLM performance improves with scale – more parameters, more and better training data, more training runs and more computation. While GPT2 boasted some 1.5 billion parameters, subsequent models have grown by orders of magnitude, with GPT4 reportedly incorporating 1 trillion such variables. The massive open data ecosystem of the internet has played a fundamental role in the development of these models. The amount of compute dedicated to training these models has also increased sharply, driven by advances in silicon innovation. Finally, breakthroughs in software and model architecture design drive and accelerate improvements.
While China had matched or exceeded the US’s historic lead in many fields of traditional AI research, the overwhelming majority of innovations in generative AI today are coming from the US, and China faces an uphill battle in training LLMs, for now.
As with past technological revolutions, the nature of different governance systems can enhance or impede progress. Open societies like the US worry about AI risks, including the accuracy of LLM outputs and hallucinations, a phenomenon wherein an LLM provides inaccurate information based on the perception of patterns that do not exist or are otherwise faulty. But they do not let those concerns halt progress. Meanwhile, closed societies, including China, have different worries, particularly about their ability to control domestic speech and content that may not be state-approved, and their capacity to advance what many analysts describe as Beijing’s “discourse power,” a way to shape global narratives. AI systems are unpredictable, and their black-box structure features inputs that are often invisible and outputs that cannot be determined by government officials and censors. These are particular concerns for closed societies, and have led the Chinese state to impose restrictions that may limit innovation, hold back incumbents, and deter new entrants and entrepreneurs from engaging with this technology.
For example, Beijing proposed new rules in April 2023 for developers and deployers of generative AI, including a requirement that “content generated using generative AI shall embody the Core Socialist Values and must not incite subversion of national sovereignty or the overturn of the socialist system.” The Party’s concern was therefore not about inaccurate information or hallucinations, but about potentially truthful outputs that may not conform to a Beijing-endorsed position. While the Chinese government has since issued somewhat less restrictive regulations, pushes for political control over technological developments highlight self-imposed challenges to AI development. As a result, Chinese LLMs are often trained on smaller, more restricted data sets than their Western counterparts. Censorship regimes may also compromise or bias access to and the production of data. And they pose even greater engineering challenges regarding moderation of non-deterministic outputs vs. democratic counterparts.
In addition to models, in recent years, the AI competition between the US and China has focused on hardware, as the clearest path to greater compute is via access to high-performance graphics processing units (GPUs). While China has a robust domestic semiconductor industry, China’s vulnerability in the “chip wars,” the competition over the microchip technologies that power AI, was exposed on October 7, 2022, by a set of US-led export controls on high-end semiconductors and related technologies, which the US coordinated with the Netherlands and Japan. These export controls follow a strategy described as “small yard, high fence,” wherein a limited number of national-security sensitive technologies are protected through strict measures.
While these US-led export controls have marked a clear turning point in US-China technology competition, there have been challenges to enforcement, including due to the need to coordinate with international partners over complex, global supply chains. To tighten enforcement, on October 17, 2023, the US released updated export controls on advanced semiconductor technology, specifically targeting chips and equipment essential for artificial intelligence development, including restrictions on high-performance chips and advanced lithography tools.
Beijing has responded to the US-led controls with its own export controls, including on critical minerals such as germanium, gallium, and more recently graphite, areas where China has a historic supply-chain advantage. More significantly, Beijing increased its focus on domestic technological development and self-reliance. As the US-China Economic and Security Review Commission reported, Chinese companies “surged their orders for foreign semiconductor manufacturing technology in 2023, capitalizing on the roughly eight-month lag between when the Dutch government announced its intent to place controls on exports to China and its implementation.”
Despite US-led export controls, China’s progress in hardware has continued, if at a slower pace. In September, the Chinese state-owned Semiconductor Manufacturing International Company (SMIC) and a Huawei subsidiary named HiSilicon demonstrated the capability to produce hardware comparable to Nvidia's A100 chip, with the fabrication of a 7-nm chip using foreign-made equipment already present in-country. This chip is shipping in Huawei’s latest smartphone, the Mate 60 Pro, which scholar Chris Miller has described as “the most ‘Chinese’ advanced smartphone ever made” because “the phone’s primary 7-nm processor [and] many of the phone’s auxiliary chips are homegrown, including the Bluetooth, WiFi and power management chips.”
The Mate 60 Pro was a significant breakthrough that showed the shortfalls of existing export controls and the capabilities of China’s semiconductor sector. While China remains two generations behind the world’s most cutting-edge chips, its technology ecosystem is a world leader in many fields, partially due to its robust domestic industries, strengths in data, and significant domestic talent pipeline. However, serious questions remain about the depth of China’s technological ecosystem, its ability to achieve comparable results without further international imports, and its capacity to scale production at acceptable costs, given the challenges that export control impose.
The chip wars have significance not only for geopolitics, but also for global markets. They have reshaped the movement of goods and intellectual capital, and major chipmakers like Nvidia have attempted to operate within the new paradigm by releasing versions of their products targeted for Chinese markets that are as advanced as possible, given the restrictions. The results have been clear: US semiconductor exports to China dropped by 51%, from $6.4 billion to $3.1 billion, in the first eight months of 2023 compared to the same period in 2022.
Meanwhile, the US is working to friendshore and onshore semiconductor supply chains. The CHIPS and Science Act introduced $39 billion in incentives for domestic chip production, and this funding includes national-security conditions, such as restricting recipients of federal dollars from involvement in the “material expansion” of “semiconductor manufacturing capacity” in a “foreign country of concern” (e.g., China, Russia, Iran, or North Korea). Such programs aim to give the US a relative edge and, as White House National Security Advisor Jake Sullivan stated, “as large of a lead as possible” in critical technologies.
But leadership in AI does not come primarily from state-led initiatives. For countries to lead in AI, they need national strategies that foster and direct innovation, as well as world-leading AI companies and research institutions. The generative AI ecosystem will empower incumbent enterprises and also likely define the next generation of Big Tech companies. Private AI investment globally is considerable and growing, and is forecast to increase to more than $160 billion by 2025, according to Goldman Sachs Research. However, sustained growth and innovation requires a system that promotes property rights and entrepreneurship, and that provides predictable rules of the road to startups and mega-cap technology companies alike. China’s crackdown on technology companies, dating back to at least 2020, and dramatic shifts like the cancellation of a leading Chinese technology company’s IPO, increases uncertainty in the non-state sector, decreasing the dynamism of China’s technology ecosystem.
The US is the preeminent AI power today. But the US-China technology competition is far from settled. We will see evolving strategies of techno-economic statecraft from both sides. In the past, China has shown a remarkable ability to overcome economic, geopolitical, and structural challenges to its position, and Beijing will continue to strive do so, as the Mate 60 Pro demonstrated. There are also potential paths of chip research and development, including advanced packaging, that provide new trajectories for improvement. For AI models, it is possible that entirely novel architectures could emerge that are not as dependent on the chips the West dominates today. Even if China remains the world’s number-two AI power, China will remain a formidable competitor to the US and to the US-led technology ecosystem.
For the foreseeable future, we expect geopolitics to increasingly driving economic decision-making in Washington and Beijing, and in capitals around the world. The US, China, and other countries are using new and old tools to advance political objectives through economic means. The table below outlines a few of the most prominent techno-economic tools shaping technology competition.
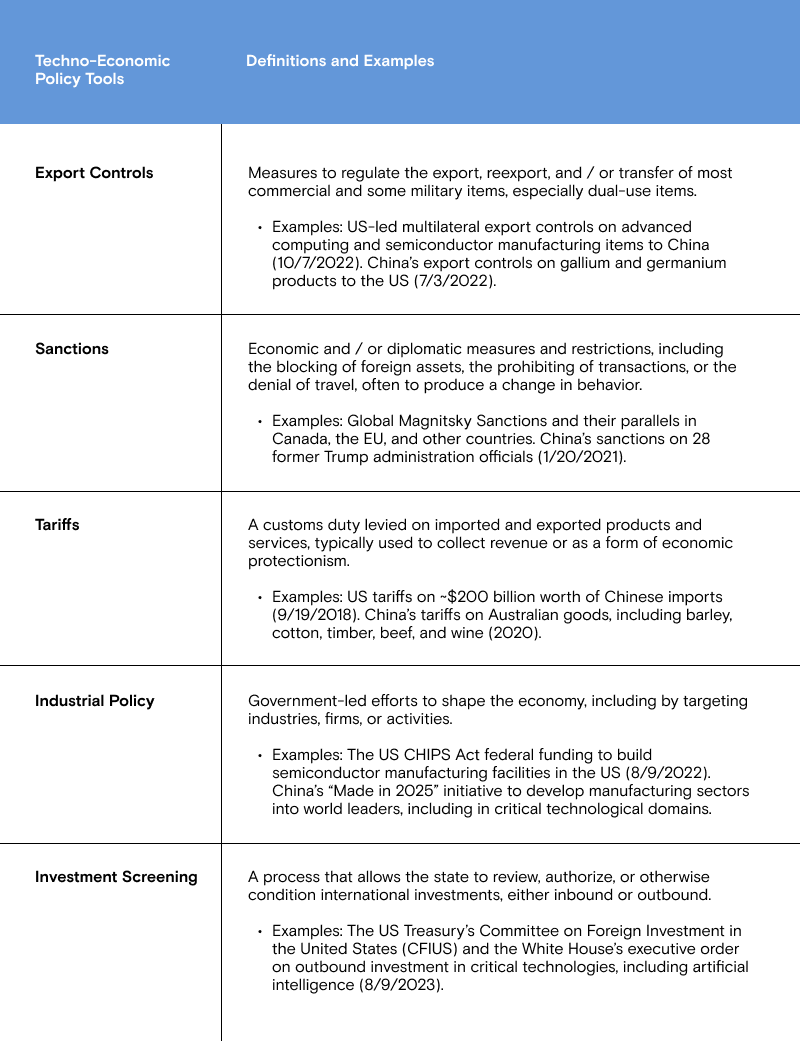
The AI capabilities of geopolitical swing states
The US and China are the world’s two AI superpowers. But they are not the only ones, and new actors are emerging. When it comes to the development and adoption of artificial intelligence, supranational organizations and non-great powers — the geopolitical swing states and blocs — have a significant ability to shape this technology, its development, and its adoption.
Geopolitical swing states and blocs have a set of differentiated capacities and interests that they bring to their AI strategies. Their power to shape the future of AI and of the emerging world order come from one or more capabilities that set them apart from other powers:
1. Economic or regulatory power to shape global technology innovation and commercialization;
2. Differentiated AI technology and talent ecosystems;
3. World-leading companies that give them control over critical chokepoints in the AI supply chain;
4. Clear national AI strategies and the capability and will to implement them and to deploy capital
The European Union
In early December, the EU Commission, Parliament, and member countries reached an agreement on the AI Act, a long-anticipated reform that is described as “the world’s first comprehensive legal framework for artificial intelligence.” With final text still pending, this agreement will likely be passed before the 2024 EU elections.
While the EU’s leadership potential on AI is limited because it has fewer, and smaller, AI companies than the US or China, the EU AI Act has significant commercial and geopolitical implications. The EU is home to 450 million inhabitants, and it is the West’s single-largest demographic bloc and a leading global commercial player. The AI Act’s regulatory framework could shape AI’s development and adoption elsewhere, as the proposed text includes extraterritorial applications on providers and users. EU regulations can alter how closely knit the transatlantic technology ecosystems can be, and other jurisdictions may adopt aspects of EU frameworks.
The proposed AI Act is far reaching, and includes rules over general-purpose AI and foundation models, which were likely added as reactions to the proliferation of tools like ChatGPT. A major component of the EU’s strategy focuses on “digital sovereignty,” an effort to control data flows and to shape the design and ethics behind AI. To do this, the EU uses a risk-focused framework in areas it deems as “prohibited,” “high-risk,” “limited,” or “minimal.” The EU aims to develop rules that would give it a “leading role in setting the global gold standard” for AI regulations, using an updated definition of AI developed by the Organization for Economic Co-operation and Development (OECD).
The AI Act makes the EU a leader on regulation, but not innovation. In 2022, 1.9 times as many AI companies were funded in the US as compared to the EU and the UK combined. The US also has seen more than four times as much private AI investment in the last decade. Meanwhile, Chinese private investment in AI-related semiconductors was 102 times higher than such investments in the UK and EU.
However, France has emerged as a leading AI power within the EU. President Emmanuel Macron stated earlier this year, “I think we are number one [in AI] in continental Europe, and we have to accelerate.” This assertion is not unfounded – Paris is home to Europe’s largest AI talent pool (though it is significantly smaller than London’s) and its AI strategy, released in 2018, focuses on developing sectors where AI will make a real difference, including health care, transportation, the environment, and security. France has invested at the national level, including EUR 1.5 billion from 2018 – 2022 and an additional EUR 500 million for AI “champions” in 2023. Most importantly, Paris is betting on open-source firms to win the technology debate and provide room to innovate within existing and pending EU regulatory frameworks.
The United Kingdom
The United Kingdom released its National AI Strategy in 2021, one year after Brexit. London’s strategy prioritizes investments and planning for the long-term needs of the AI ecosystem, support for a transition to an AI-enabled economy, and national and international governance through greater coordination mechanisms, if not the establishment of new institutions. The UK’s AI strategy can set it apart from its neighbors due to its recent separation from the EU.
The UK has a strong history of scientific discovery and AI innovation, including at its world-leading research universities like Oxford and Cambridge, and AI companies like DeepMind, now a part of Google. The UK ranks as third in private AI investment, behind only the United States and China. The UK AI market is currently valued at $21 billion and projected to scale by orders of magnitude over the next decade. The UK government has also announced $1.3 billion for supercomputing and AI research, on top of $2.8 billion in prior AI investments.
Prime Minister Rishi Sunak is working on technocratic solutions to global problems and seeks to make the post-Brexit UK a technology leader. The Prime Minister has prioritized the UK becoming the “geographical home of global AI safety regulation,” and perhaps an alternative to Brussels. The UK’s most significant advance in that regard came in November 2023, with the AI Safety Summit at Bletchley Park, which focused on managing the risks from recent advances in AI. The Summit showcased the UK’s ability to shape the global agenda by bringing together 29 governments, including notably the US and China, as well as companies from around the world, resulting in a joint declaration about AI risks that could form the basis for future collaboration.
The United Arab Emirates
The UAE has launched an ambitious AI strategy, and Abu Dhabi appointed the world’s first minister of artificial intelligence in 2017. With a focus on rapid AI innovation, the UAE established AI training programs with Oxford University and founded the Mohammed bin Zayed University of Artificial Intelligence. The UAE’s AI strategy has a different focus than its counterparts, with the goal to become “the world’s most prepared country for artificial intelligence.” The UAE may provide alternative AI ecosystems for non-great powers, and it will be a critical partner and arena of competition for the US and China.
The country’s AI preparations are extensive. UAE-based AI and cloud computing companies have recruited talent from Israel, Indonesia, China, Singapore, and elsewhere, part of a more than $10 billion investment in artificial intelligence. The country has also developed sophisticated Arabic-language open-source LLMs, including Falcon and Jais. In the absence of a domestic semiconductor manufacturing industry, the state has used its significant capital to purchase thousands of cutting-edge chips, particularly from Western countries.
The UAE’s most geopolitically significant AI strategy may be that it is reportedly positioning itself as both a complement and in some ways a competitor to both the US and China. At the same time, Beijing and Washington are competing with one another within the UAE, working to build closer technological ties with UAE-based enterprises and to persuade them to cut ties with opposing poles in today’s great-power competition. The UAE’s direction of travel will be especially important for the world’s 400 million Arabic speakers, many of whom will use its LLMs, and for much of the Global South, giving the country of 10 million people the ability to affect billions of consumers globally.
Israel
Israel, a country of just over nine million people, is a global technology leader in many fields. As author Dan Senor and Saul Singer described in their book Startup Nation, the country is now home to the world’s densest concentration of startups, especially in the cyber security and defense sectors. Israel’s technological innovation is driven by its world-leading talent, and it has long relied on its qualitative advantages to survive and thrive in a challenging region. Much of the research and innovation behind tomorrow’s cyber and defense systems is taking place today in Israel.
Necessity has driven Israel’s technological innovation, particularly in the defense and cyber domains, and both of these fields stand to be transformed by artificial intelligence, to Israel’s advantage. In some years, private investments in Israel’s cyber industry exceeds that of the European Union. In 2022, private AI investments in Israel totaled $3.2 billion, behind only the US, China, and the UK. Israel was also fourth in the number of newly funded AI companies that year. Today, Israel is using AI to cut through an “algorithmically-fueled fog of war,” using facial-recognition software to identify and track hostages taken by the terrorist group Hamas to Gaza. It is using AI technology to enhance the capabilities of its tanks, fighter aircraft, and other defense platforms.
Japan, The Netherlands, South Korea, and Taiwan
South Korea, Japan, and Taiwan are home to some of the world’s most important semiconductor design and manufacturing companies, as well as semiconductor manufacturing equipment makers. They are also located in critical geographies for global supply chains along the South China Sea and East China Sea. The world’s great powers are dependent on these countries for their own technological competitiveness.
The importance of these countries in multilateral cooperation has been clear for some time. In addition to their roles in the enforcement of export controls, in March 2022, US President Joe Biden reportedly proposed a “CHIP 4” grouping of the US and these three East Asian governments, a move seen as not only aimed at isolating Beijing, but also at creating greater supply-chain diversification and protecting companies’ intellectual property. However, the initiative has not yet made significant progress.
As artificial intelligence becomes a greater part of daily life, these countries’ geopolitical importance will continue to grow. Taiwan’s dominance of high-end semiconductor manufacturing is of particular consequence. The island nation manufactures 68% of semiconductors globally and 90% of leading-edge semiconductors necessary for advanced AI applications. No other country, and no other company, can produce high-end semiconductors in the quantity and quality of Taiwan Semiconductor Manufacturing Company (TSMC). The great powers rely on Taiwanese-made chips that power everything from smartphones to advanced weapons systems. Because of that reality, many leaders, including Taiwanese President Tsai Ing-wen, have referred to Taiwan’s semiconductor industry as its “silicon shield,” protecting it from aggression and supply-chain disruptions. However, a recent Council on Foreign Relations report argued that TSMC’s position may make potential aggression by Beijing more likely, as Beijing could have an interest in gaining control of the company and using it for geopolitical leverage.
The final country that is critical for global semiconductor supply chains is the Netherlands. The Dutch company ASML is the world’s only manufacturer of extreme ultraviolet lithography (EUV) machines, which are necessary for the ever-more sophisticated integrated circuits in semiconductor fabrication, at a cost of $330 million or more for each machine. According to Goldman Sachs Research, EUV could increase the size of the global semiconductor market from $600 billion in 2022 to $1 trillion by the end of the decade.
India
Now the world’s most populous nation, India is also one of the fastest growing major economies, and has an important and expanding role in the AI ecosystem. After its G20 presidency and the Chandrayaan-3 lunar mission, India is taking on a more ambitious global role, including in science and technology. New Delhi’s AI strategy will have ripple effects throughout the developing world and its relationship with developed economies will be critical to India’s prospects for achieving its potential in the twenty-first century.
AI could drive India’s economic development. While Goldman Sachs Research forecasts that India’s GDP will increase at a strong 6.3% this year, the country’s GDP per capita is still just $2,610. It will take sustained economic growth for the country to reach middle or high-middle income status. AI can lead to rapid productivity and economic growth, but AI adoption requires the proper institutions and technological infrastructure, and a significant portion of India's population is not yet connected to the internet.
India’s past technology successes, with a focus on lowering costs, democratizing access, and on small and medium-sized businesses, may provide a playbook for its next chapter of technological innovation. In the last few years, the country has become a hub not only for labor and outsourcing, but also for entrepreneurship and innovation. Political scientist and journalist Fareed Zakaria has pointed to the importance of the government-sponsored, data-intensive biometric identification initiative Aadhar, which provides secure IDs for more than 1.4 billion Indian citizens, and which has been described by economist Paul Romer as “the most sophisticated ID system in the world.” India’s technological capacity continues to grow, and the global share of AI publications from India increased from 1.3% in 2010 to 5.6% in 2021.
India will also shape how much of the world views the role of technology in their own foreign-policy doctrines. New Delhi’s foreign policy, and Minister of External Affairs S. Jaishankar recently wrote, “India is preparing for an era of artificial intelligence and the arrival of new tools of influence.” This is part of what he describes as India’s “multi-vector diplomacy” – for example, while China remains India’s top trading partner, New Delhi has worked to break from Beijing technologically. Most notably, India banned 59 prominent Chinese mobile apps after a deadly border skirmish with China in a disputed territory in July 2020. This move was rooted in geopolitical concerns, and the Indian Ministry of Information Technology described the apps as harmful to the “territorial integrity and sovereignty” of the Indian state. With relations between New Delhi and Beijing under strain, Prime Minister Narendra Modi and US President Joe Biden have deepened technological cooperation, introducing a joint Initiative on Critical and Emerging Technologies in January 2023, and pointing to more integration of the digital economies of the world’s oldest and largest democracies.
The emerging AI powers
Technological revolutions can change the balance of power. World leaders recognize that fact, and the AI-enabled future will shape and, to greater or lesser degrees, be shaped by every country. Most people live in a country with a national AI strategy. These strategies differ in focus, implementation, and potential, but they show that geopolitical significance in the twenty-first century is tied to leadership in artificial intelligence.
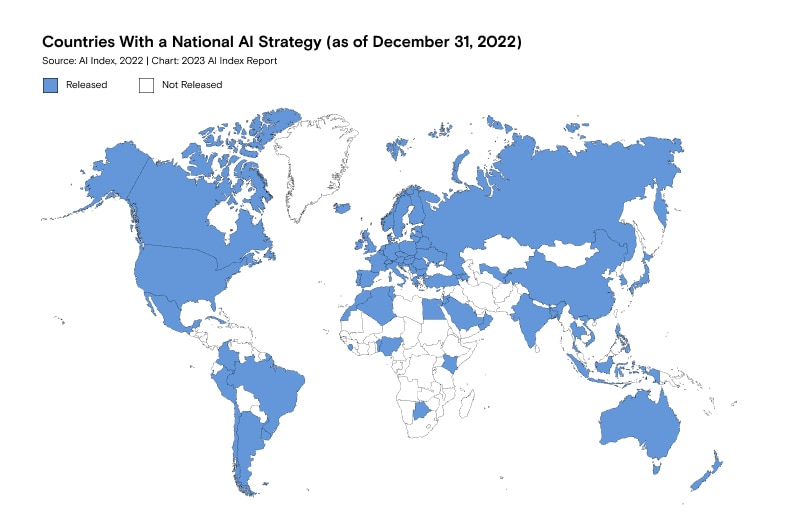
However, while nearly every country is preparing for an AI future, the effects of this technology will not be the same everywhere. According to Goldman Sachs Research, on average, developed markets, with more knowledge workers and service-sector employees, as well as greater internet access, will have greater productivity gains from AI adoption than emerging markets. According to this thesis, the top five markets that stand to benefit from productivity gains may not be the US or mainland China, but Hong Kong, Israel, Switzerland, Kuwait, and Japan. Emerging markets like India, Kenya, and Vietnam may see more modest productivity gains on a relative basis, as might be the case with mainland China.
While many past technological revolutions have followed this pattern, it is possible that the AI revolution will not. Countries whose citizens express more optimism about AI, including in emerging markets, may be more ready and willing to adopt it into their lives and institutions, accelerating the gains to be made from the AI revolution. Critically, less-developed countries may be able to leapfrog their developed peers in this technological domain.
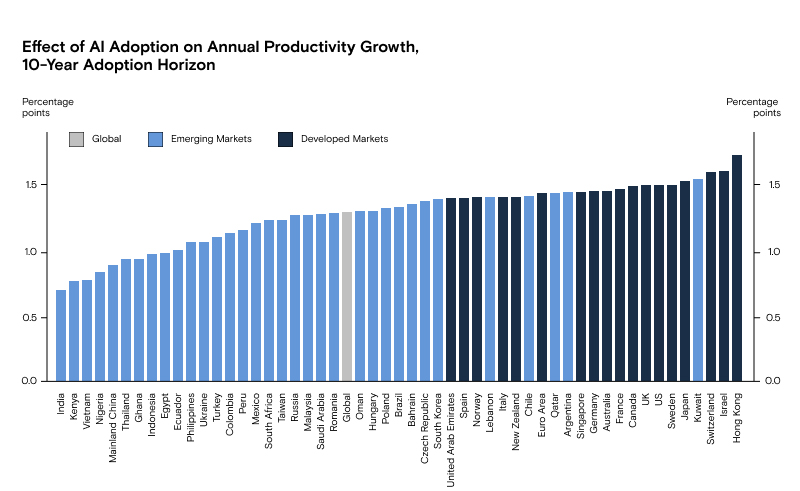
While many countries have established early leads in AI innovation and adoption, we do not yet know all of the countries that will be at the forefront of this technology. Middle Eastern nations with significant capital like Saudi Arabia and Qatar, which are focused on economic transformation through plans like Vision 2030, are working to diversify their economies into new sectors, and they have expertise in the fields of energy and logistics. Likewise, Norway, a nation of 5.4 million people with the world’s largest sovereign wealth fund, has made sustainability a key priority. These countries have many of the assets that could make them powerful AI players.
State and non-state actors may also develop new use cases, potentially increasing AI risks. Rogue nations like Iran and Russia, which do not have the capacity of global leadership in AI will use new tools to advance their malign objectives, including through misinformation, AI-enabled autonomous weapons systems, and more. North Korea, a state that has used its cyber capabilities for everything from counterfeiting to hacks of governments and companies alike may see those capabilities enhanced by AI. Generative AI is also rapidly decreasing the cost and increasing the quality of “deep fakes” and other tools for dis- and misinformation. Non-state actors, including extremist groups and criminal enterprises, will likely find new ways to exploit AI for their own ends in ways that have clear geopolitical and commercial externalities.
The age of AI will require large and small enterprises alike to create their own strategies to prepare for this changing reality.
The geopolitics of AI governance
At the dawn of the digital age, few countries were devising technology governance strategies. Today, governance issues are at the forefront of AI’s development and adoption. Policymakers and stakeholders around the world have called for multilateral cooperation to make good on the opportunities and to solve the challenges of this technology, particularly on issues of governance and safety. These discussions typically focus on three subjects:
- Misuse: AI's advancing capabilities are often dual-use and have the potential for both promise and peril. For example, AI that can be used as a chat interface to increase productivity or knowledge gathering can also be used to create and amplify misinformation, provide instructions for the creation of harmful weapons, or for cyberattacks, market manipulation, and other malign use cases.
- Unpredictability and bias: AI algorithms, particularly in deep learning, often lack transparency and have built-in biases. This can lead to discriminatory outcomes, including potentially in law enforcement, health care, and job recruitment. The "black box" nature of these systems complicates our ability to understand and predict AI behavior, making it challenging to manage unintended consequences and ensure fairness and reliability.
- Existential risk: Many investors and experts worry about the possibility of an "intelligence explosion," where AI could create fundamental risks to human society. Among this community, there is a concern that a sufficiently advanced AI might operate with objectives misaligned with universal values of human dignity, leading to significant dangers.
Leaders are worried about AI’s effects on society and human flourishing. A noteworthy early attempt to establish international AI norms came in 2021, when UNESCO provided recommendations on AI ethics, including about the importance of human oversight. A gathering of 193 member states signed the document, but while the recommendations were in line with other international initiatives, there are limits to what they can achieve. The UNESCO recommendations are not legally binding, they came before breakthroughs like ChatGPT, and significant differences remain among signatories.
Multiple AI governance initiatives have been proposed and established since that 2021 UNESCO program. The UK’s Bletchley Park Summit in 2023 was a milestone in international AI cooperation. The EU is working to find consensus among European nations. The US-EU Trade and Technology Council has prioritized cooperation on generative artificial intelligence. And the United Nations has launched an AI advisory body. But the most significant multilateral effort on AI governance may be the G7-led Hiroshima Process. Efforts among likeminded countries that are not bound by geography and include both the US and Japan are, in our view, more likely to reach tangible outcomes than disparate efforts that involve parties with major differences.
But the success of such governance efforts is still unclear. AI presents significant challenges that likely make comprehensive global governance unrealistic, especially at a time of intense geopolitical competition. And the stakeholders in the public and private sectors are often too widespread, the technology too diffuse, and the incentives to out-innovate and outcompete rivals too strong for meaningful and enforceable global norms to be agreed upon, at least in the short term, let alone the kind of “pause” that some experts advocated for in 2022.
Rather than a pause, some experts have pushed for AI non-proliferation measures akin to the arms-control measures that govern technologies such as nuclear weapons. While there are analogies between these two era-defining technologies, AI is different in kind from nuclear weapons, including because the leading edge of innovation is coming from companies, not countries, and it is being made available cheaply not only to governments but to non-state actors. In addition, nuclear arms-control agreements have not always achieved the success that many of their proponents hoped – when the International Atomic Energy Agency was founded in 1957, there were three nuclear powers (the US, the USSR, and the UK); today, there are likely nine nuclear powers that possess an estimated 12,500 nuclear warheads.
Despite the inherent obstacles, there are promising avenues for more issue-specific international cooperation among likeminded partners. Distrust of Chinese-made high technology systems, particularly on issues like data privacy, sovereignty, and human rights, have led to policy breakthroughs, including initiatives like the US-led Clean Network, which focused on 5G. One avenue for such cooperation on AI would be a grouping of technologically-advanced democracies with a limited number of key participants, a T-12 of techno-democratic cooperation. Another proposal by a prominent group of experts is an AI equivalent of the International Panel on Climate Change, a realistic and measured approach to “regularly and impartially evaluate the state of AI, its risks, potential impacts and estimated timelines.” Such an effort would be particularly valuable given the rapidly-evolving nature of this technology and the need to keep policymakers up to date.
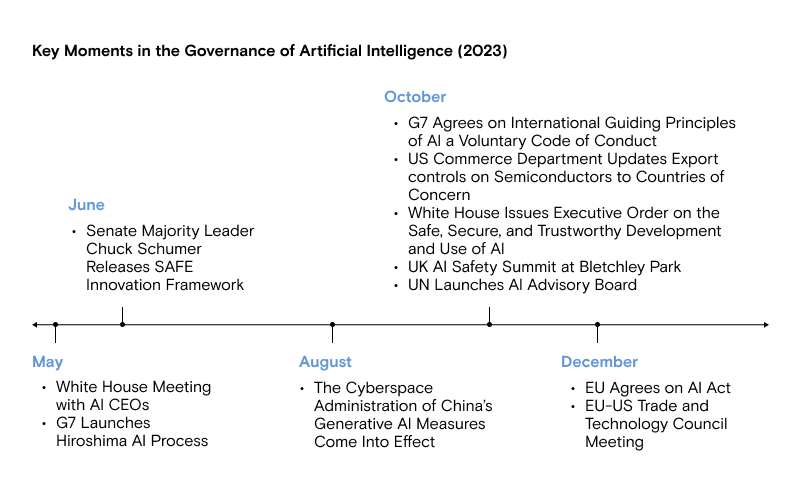
How technological development drives geopolitics
Today’s branches of generative AI represent a novel approach, but they did not develop overnight. The foundations of machine learning were laid more than 70 years ago, and the mathematician Alan Turing introduced his “imitation game,” the Turing Test, in 1950. The most significant scientific breakthrough behind LLMs came in a Google research paper published in 2017. By the time ChatGPT made its public debut in 2022, technologists were already working on the next generation of frontier models, which are more advanced and have orders of magnitude more parameters than GPT-3.
However, progress and adoption should not be taken as a given – while the technology is advancing at extraordinary rates, there have been “AI winters” in the past, and there will likely be significant pushback to AI adoption in many fields. Moreover, there is healthy debate about the sustainability of this current surge in generative AI model improvement. And while the rise of LLMs meaningfully advanced the state of the art in AI, new and even more powerful paradigms will likely emerge. To understand AI’s commercial and geopolitical significance, it is important to consider how the technology might progress. As the focus of AI shifts from pattern recognition to generation and extrapolation, so too will the arenas of technological competition.
In our view, the most important and clear emerging capabilities for generative artificial intelligence are multimodality, value engineering, and generative agency.
- Multimodality: Earlier generative AI systems were limited to processing single modalities, such as text or images. Today’s cutting-edge models, in contrast, are increasingly able to operate across diverse forms of data, such as voice and videos, synthesizing multiple communication modalities simultaneously. Multimodal AI systems will offer more holistic pattern recognition and performance – and they will greatly expand the use cases and value-proposition of these models, enabling them to approximate the many modes of human reasoning more closely.
- Value Engineering: To date, most of the research efforts in generative AI have been focused on advancing model capabilities – driving greater model scale, more data, more compute, and, ultimately, more costs. Recently, many researchers and practitioners have shifted to innovations that drive increased efficiency, including techniques such as distillation, quantization, token-dropping and efforts to find more optimal scaling relationships in the interplay of parameter, data, and computation levels. This so-called "value engineering” can drive democratization while making AI suitable for a wider variety of use cases.
- Generative Agency: Early generative AI models typically operated in isolation, performing next token prediction and answering questions based on their training data. Increasingly, these models are connected via application programming interfaces (APIs) or other means. This shift has enabled models to improve performance by expanding their capabilities and using additional resources such as calculators, domain-specific models or e-commerce websites. Associated innovations may allow models to incorporate more long-term memory functionality. Taken together, these innovations point toward more capable and persistent applications. These advances may create the possibility for intelligent agents that understand human preferences and learning styles and are able to execute complex, multi-step instructions that range far beyond the span of their own systems.
These developments will create new areas of debate, including about AI norms and values. There will be questions about adoption and deployment, as well as risk tolerance from country to country, industry to industry, and company to company. But the incentives point toward the greater integration of AI into daily life.
The key debate: scale up or scale down?
Different players will adopt generative AI in different ways. Geopolitically, the pivotal question will be whether adoption trends toward “scaled-up” or “scaled-down” models. Many of the most important debates about access and control of AI systems are downstream of the scale-up vs. scale-down debate, including the debate about open-source vs. closed-source AI. Debates around AI safety, content moderation, and even the effectiveness of export controls on semiconductors are all heavily impacted by this question.
The case for scale up: Under this scenario, most use cases will be dominated by ever-larger and more powerful general-purpose AI models. These models will demand significant compute resources for development, implying a market structure analogous to semiconductor fabrication, wherein only a small number of players can build the most advanced systems and have outsized economic and geopolitical significance.
The case for a scale-up trajectory is clear. In the past decade, scaling up machine learning models has consistently yielded significant performance improvements. This trajectory should be the default assumption, but it is not guaranteed.
The case for scale down: Under this scenario, most use cases will be satisfied by relatively smaller AI models that are fine-tuned for specific tasks. If this trend takes hold, these models will likely democratize and commoditize access to most market-ready AI use cases, enabling a broad range of entities to develop and deploy AI solutions for their specific needs.
There are many reasons to believe that the scale-down trajectory could be the future of AI development. First, existing smaller models are already adequate for many of today’s use cases. Ever-larger models may simply not be necessary for simple or discrete tasks, including image and code generation. Second, smaller, more specialized AI models are often easier to iterate upon, leading to performance that is more tailored or nimble. Third, the existing scale-up approach could begin to yield diminishing returns. Today, most text generation models focus on predicting the next word in a sequence, but leading AI researcher Yann Lecun believes that these models will reach a plateau in terms of the amount of advanced "reasoning" they can provide. And fourth, the task of sourcing new, valuable data is getting more difficult, as most of the open web has already been mined for training data, and as concerns over the use of content without explicit permissions are rising.
The future of AI governance
The development of generative AI technology will in turn shape the future of AI governance. While the precise direction of these AI system remains unknowable, we are beginning to see how different courses present enormous opportunities and risks.
- Governance under scale-up (closed-source): In the scale-up scenario, the creation and use of generative AI will require enormous data centers filled with powerful and expensive GPUs, providing clear chokepoints. This will shape governance and the enforcement of norms in AI development, including through oversight and rules on a limited number of entities, such as national champion companies. Barriers to entry, including capital, talent, and the ability to work within regulatory frameworks will be easier to define and will favor incumbents.
- Governance under scale-down (open source): Decentralized AI development would result in a more diverse innovation landscape, allowing a greater number of actors to deploy powerful models, provided they have the necessary infrastructure, including semiconductors. While this approach would increase the risk of misuse, it would mitigate the risk of power concentration. Meanwhile, smaller, more purpose-built models can be more predictable and auditable, increasing the efficacy of AI safety measures. With so many state and non-state actors having access to cutting-edge AI-enabled tools, this implies an industry structure that present considerable promise and peril as it proliferates to both trusted and malign players.
Charting the chip wars
The scale up vs. scale down debate will also shape the development and use of semiconductor technology. As the US-China Economic and Security Review Commission stated in its 2023 report to Congress, “For logic chips (semiconductor devices that perform computer calculations to power digital devices) and system memory chips (high-performance semiconductor devices that rapidly store data during computations), the degree of sophistication is measured in the width of transistors placed onto a silicon wafer, as more transistors in a smaller space can generally process more calculations.” Thus far, the main driver of AI progress has been enabled by the rapid growth in the computing power of each chip. Today’s most advanced logic chips have transistors as small as three nanometers (nm) in width, enabling tens of billions of transistors on each chip.
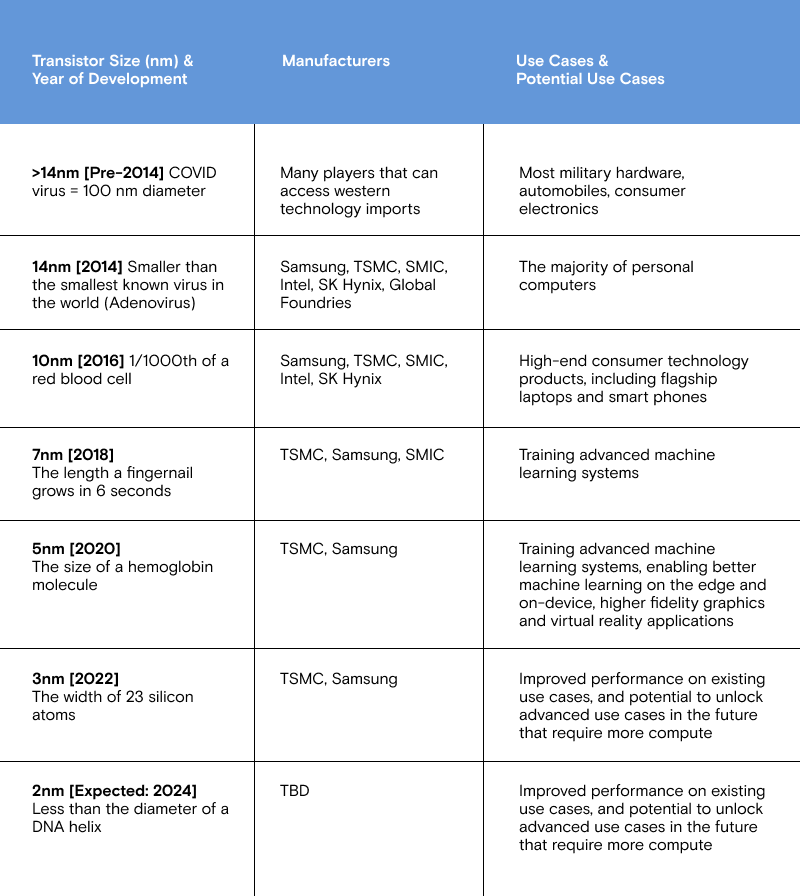
Following Moore’s Law, the processing power of today’s most advanced chips is exponentially greater than the most advanced chips of a decade ago. But while this increase has yielded greater processing speed and performance, there may be a point where physical constraints limit the possibility for further advances, or where there are diminishing returns to those advances, including for consumer applications and geopolitically-relevant use cases.
Hardware has taken on new importance for technological development and geopolitical competition. For now, a US-led strategy of semiconductor supply chain de-risking and technology competition, coordinated with Japan and the Netherlands, as well as other countries, has focused on export controls, which aim to inhibit China’s access to advanced semiconductor technologies beyond the 16nm threshold, a level that the US first achieved in 2014.
If the benefits of scaling up AI models reach the point of diminishing returns, then the restrictions on China’s AI development may become less effective. For example, in the domain of military hardware, legacy, trailing-edge chips are often sufficient for today’s strategic use cases, including missile guidance systems. When it comes to AI, models that were once cutting-edge, but are no longer, could still accomplish many specific product and use case goals. Under this scenario, export controls on more advanced chips might not yield a significant impact, including in the national-security domain. However, as weapons systems continue to become more advanced, it is possible that higher-end chips will become even more important in the military domain.
Overall, semiconductor export controls’ importance will increase as future AI-enabled capabilities require greater computing power than legacy chips are able to provide. The US and its partners’ ability to cooperate on controls around these chokepoint technologies will strengthen their relative positions. It is possible that new measures will be introduced, including “cloud computing end-use rules,” which have been proposed by US congressional committees. And while we do not yet know the limits of existing computing technology, history teaches us that the possibilities of the digital world are unlocked by ever-more potent computing power – and, undoubtedly, countries with differentiated access and control of cutting-edge computing power will continue to wield outsized geopolitical influence.
AI and commerce
Generative AI represents a fundamental technological breakthrough. But its potential use cases, and what they could mean for business, are what have captured investors’ imaginations. Projections for the commercial impact of generative AI span the conservative to the paradigm-shifting. Goldman Sachs Research estimates a baseline case in which the widespread adoption of AI could contribute 1.5% in annual productivity growth over a ten-year period, lifting global GDP by nearly $7 trillion (roughly the size of the economies of Japan and Canada combined). The upside case carries a remarkable 2.9% total uplift, and even greater increases to global GDP. While top-line estimates of AI’s global impact are eye-catching, these outcomes are not guaranteed. The AI-enabled effects on commerce will be shaped by four key components: energy, computation, data, and models. The direction of travel for generative AI in each of these areas – or layers – is subject to broad speculation and will be determined by today’s ongoing debates.
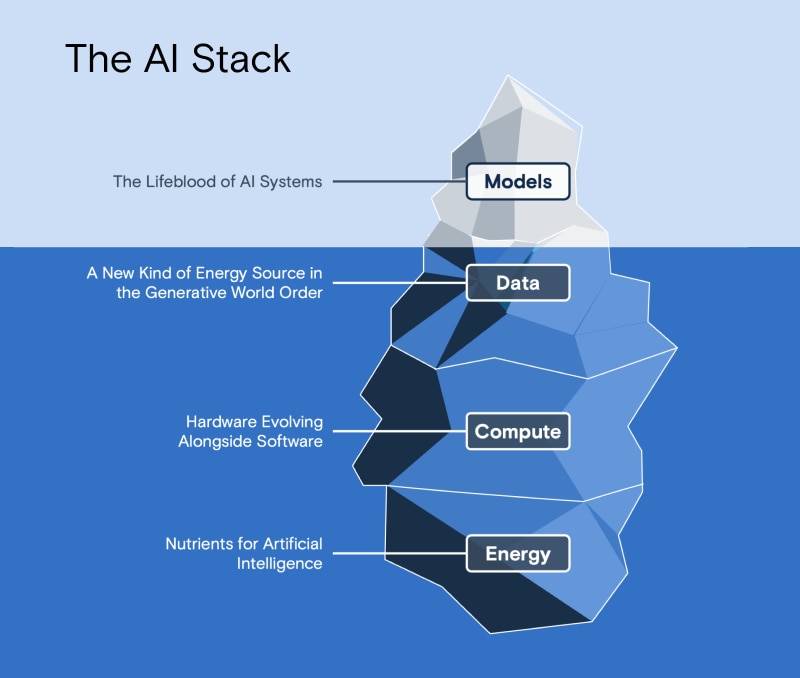
Energy
While energy is the prerequisite for running any computing system, it is especially critical in training and running AI systems.
Today’s computing ecosystem – and, by extension, its energy consumption – is centralized in large cloud data centers. While these data centers are expansive, they are surprisingly energy efficient. From 2010 to 2018 global computing output in data centers jumped six-fold while energy consumption rose only 6%. This relative efficiency reflects a concerted effort by cloud computing players and data center operators to optimize energy usage and performance.
The rise of LLMs is changing the core criteria for data centers. GPUs have a significantly higher maximum energy consumption rate compared to computing processing units (CPUs), the chips that power traditional data centers. This gap is only widening as GPUs become more sophisticated. While leading cloud providers’ newest data center chips use 60% less power than the previous generation, cutting-edge GPUs have increased power consumption in every successive release.
We expect GPU’s energy consumption to continue to scale. For GPUs, greater energy drives greater computation, and each new generation of larger LLMs will require ever-larger clusters of high-performance GPUs to train, driving ever-greater power needs.
Increased energy demands for data centers will create new priorities for data center operators. Tomorrow’s AI data centers will require geographies and supply chains that can guarantee the reliable supply of affordable energy, making the cost and availability of power a key factor in determining data center locations. This shift will confer an advantage on geographies with a significant supply of energy, including areas with their own energy resources, which will be able to serve as prime AI data center markets. Resource-rich geographies, even remote ones, will have an advantage that was less relevant for CPU data centers, which prioritize proximity to end-users. Market participants who can position themselves according to this shifting dynamic will be able to capture outsize value at the energy layer.
Compute
Hardware is evolving alongside software, particularly the hardware needed for compute infrastructure, including high-end semiconductors. Two emerging trajectories in AI compute infrastructure are likely to drive significant commercial impact, including today’s GPU shortages and resulting market asymmetries, as well as and shifts in AI workloads from training to inference.
Today, there is far more demand for cutting-edge GPUs than supply. Leading GPU providers have reported 250% or greater year-over-year gains in their data center segments in recent quarters, receiving multiple turns of valuation uplift from public markets. The vast supply-demand imbalance present in the market for GPUs has driven companies and countries to over reserve and stockpile capacity, conveying the geoeconomic importance of this technology and the uncertainty of the global supply chain.
Companies and countries have a variety of strategies to meet their expected future GPU demands. In many cases, AI chips are being acquired or preemptively leased, even without prima facie use cases. These actions may be in the anticipation of export controls, or simply due to the assumption that this technology will be so consequential that stockpiling is an economic or business imperative. Mass acquisition of compute capacity has exacerbated the disconnect between GPU supply and demand, creating idiosyncratic market dynamics. For instance, systems like the cloud of the last decade – with fully virtualized compute power that can “spin up" and "spin down” – can no longer operate for cutting-edge GPUs in this environment of relative compute scarcity. Until enough GPUs are produced to satisfy companies’ and countries’ expectations for today’s and for future use cases, we would expect these deep market asymmetries to persist. This will in turn create demand for more efficient compute allocation to return this market to equilibrium.
At the same time, AI workloads are shifting from training to inference. Training is the process of developing and “teaching” AI models (i.e., building an AI application). Inference is the process of putting a model’s “learning” into action and executing LLM workloads (i.e., using an AI application). Training is analogous to capital expenditure, while inference is analogous to cost of goods sold AI systems. AI systems require training before they can be run, but once the necessary infrastructure and LLMs are built (i.e., trained), they can be run (i.e., perform inference) by end-users.
The wave of investment in generative AI systems to date is extraordinary, and its pace is projected to accelerate. According to Bloomberg Intelligence, from 2020 to 2024 private-sector spending on generative AI-centric systems will have increased from $14 billion annually to $137 billion, totaling $280 billion in five years. Most of these investments have been on activities related to training new AI models, including by building new data centers, buying GPUs, and a wave of venture capital fundraising focused on compute. Assuming widespread adoption, we expect a pivot, with inference becoming the lion’s share of AI workload spend.
This shift from training to inference has the potential to alter markets, as training and inference involve different hardware and software needs. For example, inference workloads are not only far less computationally intensive vs. training, they are also far less technically sophisticated, requiring fewer detailed instructions for the physical hardware to run efficiently. Consequently, inference can be done on less-advanced platforms. More advanced hardware can run inference faster, but access to cutting-edge hardware may not block progress in the same way than it can today. These changing realities have significant geopolitical implications, including on the efficiency of existing export controls.
A world in which inference is the predominant AI workload may also alter the landscape of commercial winners and losers. Leading chip providers that rely on a software ecosystem as their “moat” in training may no longer have the same advantages in inference workloads, opening space for other chip players to take market share. An inference-forward world could drive a decentralization of compute locations, given that inference can occur further away from end users as compared to today’s typical internet workloads, which prioritize latency and bandwidth. This may require companies to begin planning for a time when new data centers are economically feasible, new chips are prioritized, and there are new greenfield opportunities for growth.
Data
Today, data is no longer merely a record of a past event, but also a kind of energy source for the creation and improvement of intelligent behavior and the nascent capability of AI to reason. As a result, data sources will be more closely studied, treated, and traded. While AI training is starting to near the limits of the raw data that the open web can provide, new proprietary sources of data continue scaling.
Data is essential across all forms of compute. But nowhere is that truer than in training and running AI systems. Each new generation of LLM has been ~10 times larger than its predecessor. Industry research has shown that the amount of data required to train models must increase 1:1 with the size of the model. To date, this requirement has not been an issue, as model developers could use an ever-large percentage of the open web as training data. However, in the next generation or two of cutting-edge LLMs, it is possible that the available data on the open web will be exhausted.
At the same time, owners of intellectual property, including publishers and content-creators, are pushing back against their content being used in AI training data, voicing concerns about intellectual property. These groups are often well-organized and well-connected, and have incentives to protect their intellectual property, including by establishing new norms and guardrails and by creating marketplaces for legal licensing of content and intellectual property, harkening back to the internet’s transition from the “Napster Era” to today’s “Spotify Era.”
As we reach the limits of publicly available data, private data will likely grow in importance. While proprietary data comes with additional concerns, including around privacy and licensing, companies will be incentivized to find solutions to increase their data pools. We anticipate this will open new commercial pathways in creating systems to buy and sell access to trusted data – for example, via new kinds of licensing agreements to use existing, trusted data to train AI systems (e.g., licensing stock imagery, social media comments, etc.).
We anticipate a new model emerging for “data rich, revenue poor” platforms. Internet businesses – especially those already struggling with monetization – may be able to unlock new revenue streams if they have sufficient high-quality data available. Many companies today may be undervalued, as traditional discounted cashflow analyses may overlook the potential for future data licensing revenue.
There are three main categories of platforms that are particularly valuable for AI services. First, platforms with massive data volumes needed for models that require broad inputs for performance. Second, platforms with limited but especially high-quality data from trustworthy sources, including sources free of toxicity or with particularly ordered and logical structures. And third, platforms with domain or task-specific data, related to a unique commercial topic, for example international tax law, or data that is well-structured for a specific use case, such as high-quality sales transcripts, which can be more effective at driving models to produce desired outputs.
These platforms will change how commercial enterprises engage with their data. No matter their size or sector, companies will consider analyzing and cataloging their data stores to assess new opportunities, including for licensing data to others and training and fine-tuning proprietary models for their own use cases.
Models
For the last year, most commercial attention has been focused on large, closed-source foundation models, such as GPT-4 and Bard, Google’s AI service. However, there has also been an explosion in the number and variety of much smaller, open-source models, such as Llama2 and Falcon. The former features extraordinary levels of language capability and world knowledge. The latter is more adaptable and well-suited to narrower, domain-specific tasks. Smaller, open-source models also promise more “edge” applications with “intelligence” natively embedded in more devices and useful in more distributed scenarios.
Smaller models (both open and closed-source) have made tremendous strides in the last year, and their proliferation would have many downstream effects. First, there may be some commoditization of foundational model AI companies, as pricing pressures force a race to the bottom. Second, there may be additional value captured at the application layer, as internet companies will be able to develop their own customized AI implementations by fine-tuning smaller models or switching between competing model providers, rather than being dependent on only a few suppliers of cutting-edge models. Third, if the scaled down model continues to progress, we will likely see a rise of vertical-specific models with hyper-relevant data as the key moat. In this paradigm, the concept “there’s an app for that” could become “there’s a model for that.”
Technological developments will also reshape the structure and purpose of models, including by enabling ever more powerful and personalized AI assistants. A trend we have been closely observing is the gradual evolution of LLMs from siloed ask-and-answer machines toward greater generative agency, wherein models increasingly initiate and coordinate more complicated prompts and tasks. While early foundation models demonstrated broad capabilities, they have generally been architecturally isolated, giving users the ability to ask questions that the models would then answer to the best of their independent capabilities, based on their training. Today, models are connected to a range of other resources via application programming interfaces, plugins, and more. This gives them the ability to access information and capabilities inherent in other models or online resources, multiplying their potential “intelligence” and precision, but also allowing them to interact more with the “real world” and execute instructions on users’ behalf. This direction, which also will necessitate the incorporation of greater and more persistent memory resources, raises the potential for highly capable, personalized, always-on AI assistants which can help us plan and execute instructions.
The rise of generative agency would affect model companies – both large and small – and incumbent internet companies in consequential ways. For model companies, scale would quickly become a distinct moat, and developers would likely seek to build sub-models, plugins and tooling for ecosystems with the most users. A positive loop of more users would lead to more sub-models and plugins, which would in turn lead to better functionality. Data would become an even greater differentiator, and agent models would seek to farm-out tasks across interoperable sub-models. At the same time, models with high quality, specialized, proprietary data may have more ability to generate usage and economic leverage vs. competing sub-models. Pricing power would dramatically increase for the select few horizontal AI agents that achieve sufficient scale.
In this scenario, incumbent internet companies would have to grapple with established profit centers and economic models coming under greater pressure. Internet businesses with differentiated moats, such as search, e-commerce, or multimedia, would need to re-think their interfaces for a world in which humans are increasingly disintermediated from direct interaction, as point-and-click user interfaces will give way to natural language navigation via AI assistants. It is likely that agent companies would capture some of this value themselves, and select incumbents would be able to reinvent their services via APIs, plugins, partnerships, and more. At the same time, other enterprises would face potentially existential pressure. Finally, generative agency may also drive a great “re-bundling” from the cloud era, in which company formation proliferated as many legacy platforms were unbundled into disparate best-of-breed tools. In the AI era, the reverse may occur, where horizontal AI agents consolidate and re-bundle services together.
What to watch
The rate of AI progress is occurring at such a speed and scale that it is impossible for any one person, company, or country to predict the future of this technology, or how it will change the way we live. But we can see how AI is already reshaping technology, commerce, and geopolitics. In this inter-AI period, we have the opportunity to direct the course of the AI-enabled future. We will witness several key milestones that will give a picture of the emerging generative world order.
In 2024, 41% of the world’s population will head to the polls, including the three most populous democracies, India, the United States, and Indonesia. With significant elections also occurring for the EU Parliament and in Taiwan, at the end of next year we will better understand how AI is both strengthening and undermining democratic societies. With democracies facing challenges on multiple fronts – including ongoing wars in Ukraine and the Middle East – we will also have a better picture of how AI is changing the character of war and how liberal societies defend themselves. As former US undersecretary of defense Michele Flournoy wrote, “In the future, Americans can expect AI to change how the United States and its adversaries fight on the battlefield.” That future is coming faster than many think, including AI-enabled unmanned surface, subsurface, and aerial vehicles and “intelligent” ballistic missiles, as well as other weapons systems that can identify, track, and attack their own targets, potentially without humans in the loop.
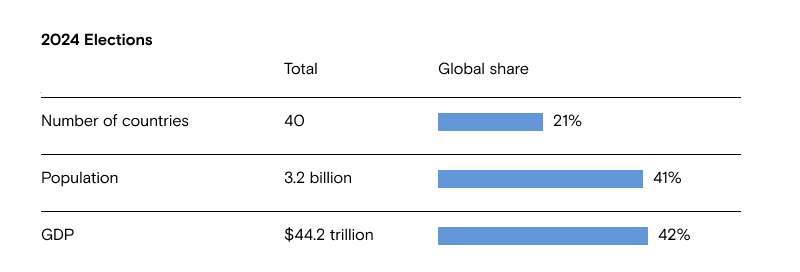
In the medium term, AI will reshape scientific discovery and reengineer globalization. AI is already enhancing scientific breakthroughs, including data-driven strategies to speed up Covid-19 vaccine research and development. AI will accelerate the scientific method across the board, and in the health-care field will provide new methods for drug discovery, disease detection, and clinical trial facilitation. Alongside the historic benefits, there could be new and more dangerous use cases, including biological and other forms of weaponry. With geopolitical competition on the rise, AI could accelerate the fragmentation between the US and Chinese technology ecosystems, and other countries may have to choose between the two competitors as they seek to protect their digital sovereignty and interests.
The pervasiveness and scale of AI will mean that its effects will be felt in every part of society. Many observers worry about job loss, as AI has the potential to automate 25% of labor tasks in advanced economies and 10 – 20% in emerging economies. But those fears should be balanced with a grounding in the history of past technological revolutions and in a focus on the new opportunities that AI will unlock. On net, technological innovation drives economic progress, improves social welfare, increases workers’ wages and income, strengthens demand, and creates new jobs and industries that our ancestors could not have imagined. As economist David Autor reported, “Roughly 60% of employment in 2018 is found in job titles that did not exist in 1940.”
There is no turning back the clock on AI. The most important question will be how we will live in a new technological era, when a human-like intelligence, perhaps one day an artificial general intelligence, is a part of our world. Today, we have the power to choose what that future will look like. This period will not be repeated. It’s up to human beings to choose wisely.
Related reading from the Office of Applied Innovation:
The rise of geopolitical swing states
Resource realism: The geopolitics of critical mineral supply chains
The global credibility gap: Assessing underperformance and overreach in today’s geopolitics
This document has been prepared by the Office of Applied Innovation at Goldman Sachs and is not a product of Goldman Sachs Global Investment Research. This document should not be used as a basis for trading in the securities or loans of the companies named herein or for any other investment decision. This document should not be construed as consisting of investment advice. This material is intended only to facilitate discussions with Goldman Sachs and is not intended to be used as a general guide to investing, or as source of any specific investment recommendations. Certain information contained here may constitute “forward-looking statements” and there is no guarantee that these results will be achieved. Goldman Sachs has no obligation to provide any updates or changes to the information herein. Goldman Sachs is not providing any financial, economic, legal, accounting, or tax advice or recommendations. This material does not purport to contain a comprehensive overview of Goldman Sachs products and offering and may differ from the views and opinions of other departments or divisions of Goldman Sachs and its affiliates.